(FREE) The Emergence of Large Language Models in Financial Markets: A Strategic Imperative for Hedge Funds and Proprietary Trading
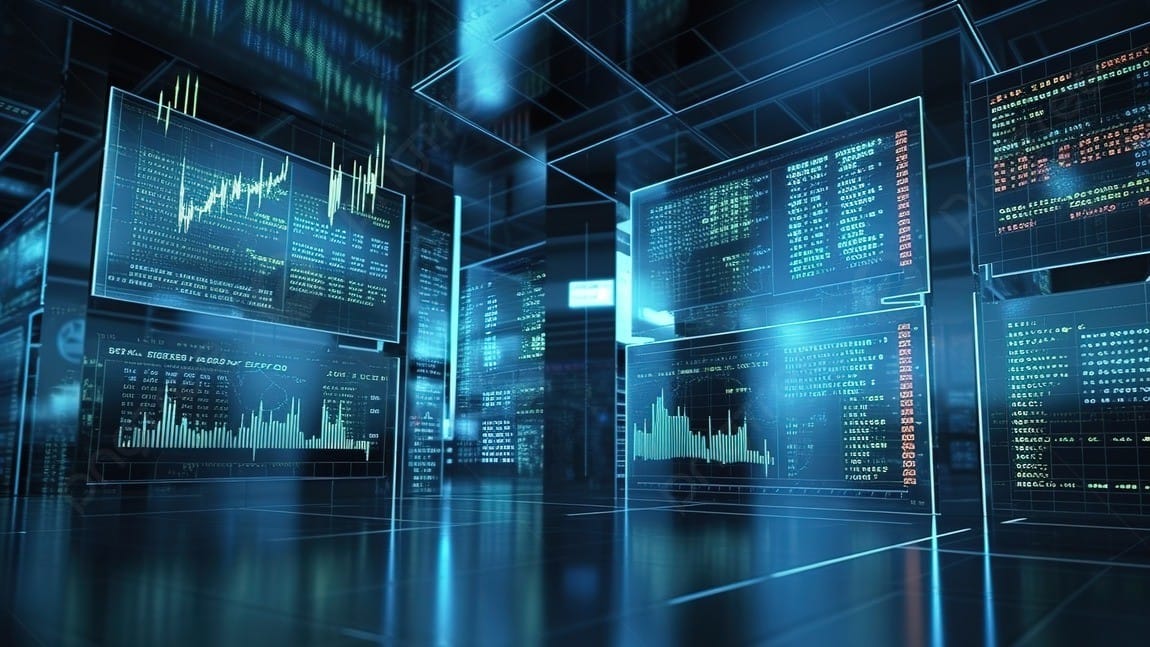
In today’s dynamic financial landscape, the rapid adoption of advanced technologies has been a consistent hallmark—from high-frequency trading to cutting-edge machine learning systems. Now, sophisticated artificial intelligence, particularly Large Language Models (LLMs), is reshaping the industry. Developed by leaders such as OpenAI, Google, and xAI, these models excel in processing vast, unstructured datasets—from news and earnings reports to social media sentiment—providing unparalleled insights and strategic advantages across both traditional finance and cryptocurrency markets.
Integrating LLMs into Financial Strategy
LLMs harness transformer architectures trained on extensive textual data, enabling them to generate nuanced human-like language and extract key intelligence from diverse information sources. In an industry where information asymmetry can define profitability, these models empower firms to consolidate and analyze disparate data streams at scale, offering a decisive edge in market sentiment analysis, price movement forecasting, trade strategy automation, and risk management.
Hedge Funds: Enhancing Alpha Through Intelligent Analytics
For hedge funds, where proprietary data analysis is crucial, LLMs are becoming indispensable. Their ability to perform real-time sentiment analysis across platforms such as Bloomberg, Reuters, and even unconventional sources like social media, provides immediate and refined insights into market reactions—be it in response to a Federal Reserve announcement or regulatory shifts in the crypto sphere.
Beyond sentiment, these models augment traditional fundamental analysis by parsing through earnings call transcripts, SEC filings, and nuanced management commentary. Such granular extraction of hidden insights or subtle strategic shifts helps identify latent risks and opportunities, complementing the quantitative models employed by industry titans like Two Sigma and Renaissance Technologies.
Moreover, LLMs facilitate the generation of novel trade ideas. By assimilating historical market data, macroeconomic indicators, and real-time news, they help formulate hypotheses—such as identifying short positions based on subtle language cues during corporate disclosures—while still necessitating expert human oversight for final execution.
Proprietary Trading: Speed, Precision, and Enhanced Risk Profiling
Proprietary trading firms, operating with significant capital at risk, are leveraging LLMs to refine high-frequency trading (HFT) and market-making algorithms. In traditional markets, these models process order book dynamics, economic reports, and real-time news, enabling rapid adjustments to trading algorithms within microseconds. Their utility is even more pronounced in the cryptocurrency domain, where volatility is high and data streams are less structured. Firms active in both spheres, like Jump Trading and DRW, are reportedly using LLMs to interpret blockchain events—such as large-scale wallet movements or DeFi protocol updates—to pre-empt market moves.
Additionally, LLMs contribute significantly to risk management by simulating stress scenarios based on historical and current data. This predictive analysis is crucial for stress-testing portfolios against potential black swan events, ranging from market crashes to cyber breaches in crypto exchanges.
Transformative Potential in Crypto Markets
The inherently decentralized and innovative nature of the cryptocurrency space makes it an ideal environment for LLM deployment. These models assist in deciphering technical whitepapers, scrutinizing smart contracts, and flagging ambiguous language that may indicate systemic vulnerabilities. Such capabilities not only protect traders from potential pitfalls but also unearth arbitrage opportunities by correlating cross-exchange price discrepancies with real-time sentiment analysis.
Furthermore, the continuous, fatigue-free operation of LLMs offers a strategic advantage in the 24/7 crypto market, enabling more agile and precise trading decisions.
Bridging TradFi and Modern Analytics
In traditional finance, institutions such as Goldman Sachs and JPMorgan are integrating LLMs within their research divisions to distill vast amounts of financial literature into actionable insights. Portfolio managers leverage these models to backtest strategies over extended market cycles, while compliance teams use them to monitor communications for potential regulatory issues. However, the transition is not without challenges, as legacy systems built around structured data must be reconciled with the unstructured, probabilistic outputs of LLMs.
Navigating Challenges and Future Prospects
While the advantages of LLMs are substantial, their deployment is not devoid of challenges. The opaque nature of these “black box” models raises critical concerns regarding interpretability, especially when substantial capital is at stake. Overreliance on similar signals from multiple firms could exacerbate market volatility through herd behavior. Furthermore, the quality of input data remains a pivotal issue—noisy or biased datasets can skew outputs, leading to suboptimal trading decisions.
Regulatory bodies, including the SEC and CFTC, are beginning to scrutinize the implications of AI-driven trading practices, necessitating a balanced approach that incorporates both technological innovation and robust oversight.
Looking forward, the evolution of multimodal AI—which integrates text, numerical data, images, and even behavioral cues—promises to further blur the lines between human judgment and machine-driven analytics. In crypto markets, the possibility of fully autonomous trading bots executing complex strategies is on the horizon, while in traditional finance, LLMs may democratize access to sophisticated analytical tools, reshaping the competitive landscape.
Ultimately, the firms that strategically harness the power of LLMs—integrating their speed and analytical prowess with prudent human oversight—will be well-positioned to navigate the increasingly complex financial markets of tomorrow.
The content provided on CryptoSazz is for informational and educational purposes only and does not constitute financial, investment, trading, or other advice. Nothing on this site is a recommendation or solicitation to buy or sell any financial asset or to adopt any investment strategy.
CryptoSazz may discuss market trends, macroeconomic developments, and quantitative trading models; however, these are intended solely to share insights and analysis and are not tailored to your specific financial situation or investment objectives.
While we strive to ensure the accuracy and timeliness of the information presented, CryptoSazz makes no warranties or representations regarding its completeness or reliability. All opinions expressed are subject to change without notice.
Cryptocurrencies, DeFi protocols, and digital assets involve significant risk, including the potential loss of principal. You should conduct your own research and consult a qualified financial advisor before making any investment decisions.
Past performance is not indicative of future results.